Debunking Fake News
Checking the Authenticity of Media Data
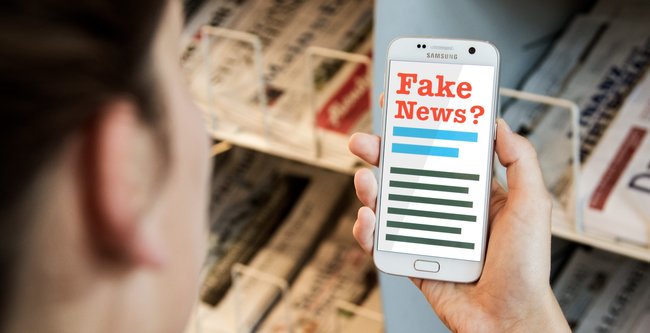
Digital multimedia data are a pillar of fact-based reporting in today’s media world. However, only simple means are necessary to tamper with or modify such media data. Fraunhofer SIT is offering a wide portfolio of services and tools to detect manipulations. One challenge in doing so is the efficient inspection of large media data sets, as they often occur in forensic investigations. In addition, media data often contain many invisible traces.
Audio Manipulations
Audio data can be easily manipulated using standard audio editing software. Especially the cutting and copying of passages is possible without much previous knowledge. However, these processes do leave traces - room reverberation and background noise are examples here. But also the frequency response of a microphone can leave characteristic traces during recordings. Cutting and reusing audio can also be detected by robust hash methods that detect identical passages in the audio stream. Machine learning methods are already capable of learning the characteristics of individual people's voices and using them to make arbitrary statements with them. Recognition is possible if these processes leave traces such as interpolation of sound fragments or an adjustment of dynamic behavior.
Image Manipulations
Images are easy to manipulate, but each change leaves its trace. Our processes and algorithms recognize these discrepancies and indicate tampering. We also examine the meta data, verify the cameras a data source (“camera ballistics“) and search for comparative image material in the Internet. Further research leads us to evidence of anti-forensics, i. e. attempts to obscure the manipulations.
https://www.youtube.com/watch?v=U17v7-Acx04
Video Manipulation
To detect video manipulations the material is first checked and correlated with regard to its meta data. Do the segments that should be temporally aligned really match? Is there a temporal offset or gap? Do segments repeat? The individual elements may be analyzed as well. This allows checking whether contents exhibit unusual transitions caused by cuts. To do this, we review the noise of the data and behavior of the sound, for example.
https://www.sit.fraunhofer.de/de/itforensics/analyse-und-verbesserung-it-forensischer-werkzeuge/
Fake News
Disinformation (fake news) refer to publications that are demonstrably factually wrong or misleading and published with a manipulative intent. In the DORIAN project we are developing semi-automated to fully automated detection techniques for fake news.